Background
“Dad, you have to try it, don’t you?’ Mary insists again.
Enrique doesn’t answer his daughter. What she’s implying will make him spend even more time in the hospital, to help someone in the distant future whom he will never meet, maybe. When he was 70, Enrique was diagnosed with acute myeloid leukemia (AML). As he understands it, some of his blood cells will never mature and perform the correct functions in his body. This cancer can be cured through chemotherapy in 25% of cases, but in most cases cannot be treated or reversed (Kornblau 2014). Enrique is contemplating joining a drug trial. In any given trial there is only a 10% chance that a patient will respond to the specific drug tested (Kornblau 2014). Some therapeutic drugs work for some patients, but how to match a patient to a drug is unknown; this is what the study Enrique might join is trying to figure out.
This study is using precision medicine, a revolutionary approach to medicine. Rather than a “one size fits all’ method, precision medicine determines which intervention will be effective for an individual patient based on his or her genes, environment, and lifestyle (NIH 2017). Common examples of precision medicine include receiving a blood transfusion that matches your blood type, having a customized glasses prescription, and receiving a treatment for any condition that works with your income-level and preferences. This is not a new concept; however, the approach has gained momentum in recent years due to advances in technology and genome sequencing as well as the Precision Medicine Initiative, which is a federally-funded research enterprise that aims to bring precision medicine into clinical practice (NIH 2017).
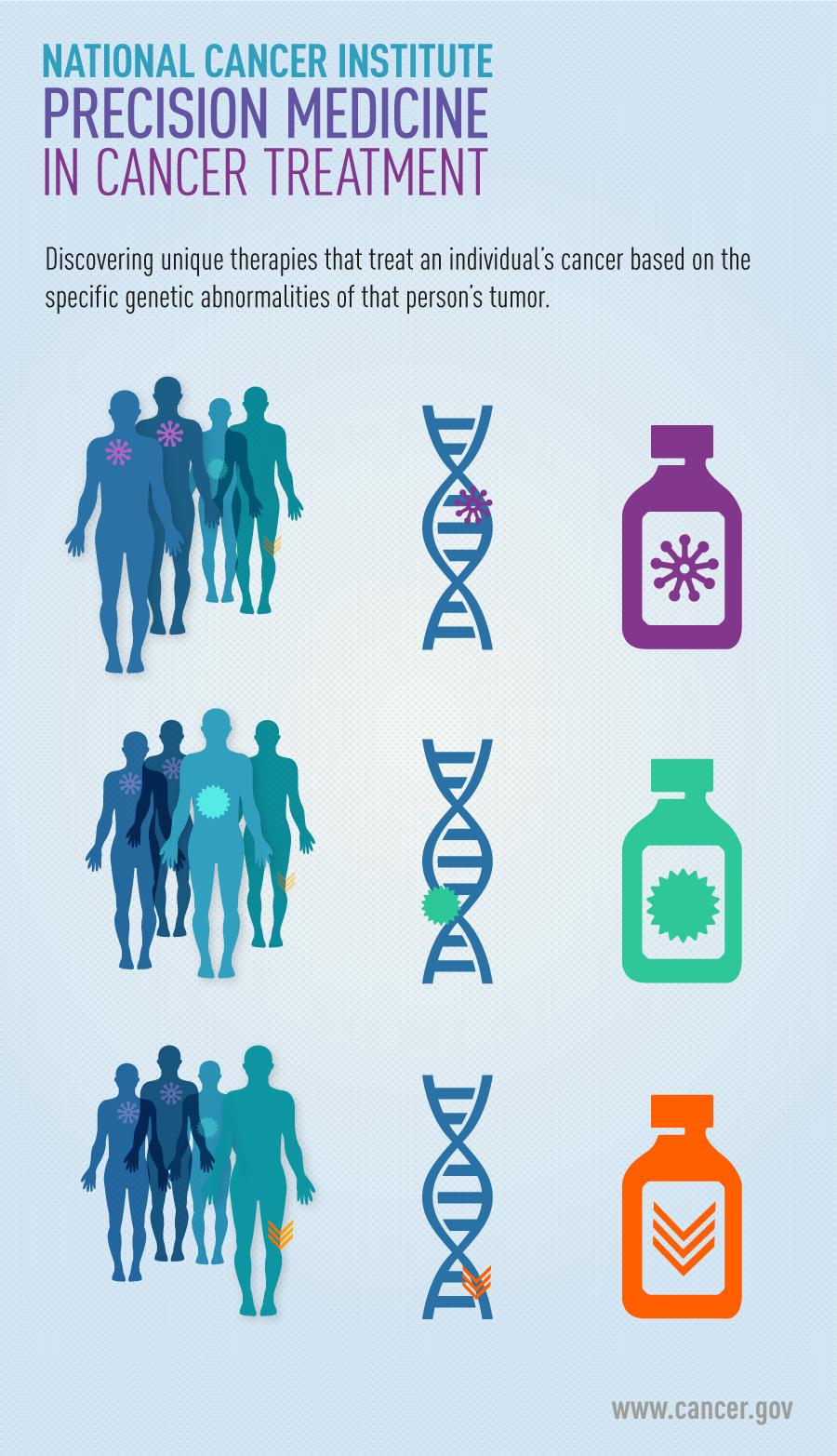

Independent companies are also investigating precision medicine in different diseases. Dialogue for Reverse Engineering Assessments and Methods, or DREAM, is a nonprofit company that creates curated datasets and crowdsources its analysis to the worldwide scientific community. Most of the time in scientific research, one team assembles and analyzes a dataset. This is a good way to find one solution, but not always a good way to find the best solution. In contrast with the old model for data analysis, crowdsourcing pits many teams of scientists against each other to discover the best solution available (Kornblau 2014).
To find the best way to match AML patients to therapies, DREAM launched the acute myeloid leukemia outcome prediction challenge. It assembled a dataset of demographic information, prior history, genetic labs, therapy, and outcomes for AML patients over four years. Then, DREAM asked researchers to create a model that could predict which therapies will be useful in which patient. This is the story of how a team of scientists used evolution to find the best model.
Central Questions
- Can evolution-informed models accurately predict development of disease in individuals?
- What are important biomarkers for cancer, specifically AML?
- How can we predict if an AML patient will be resistant to chemotherapy?
Evidence
A team headed by Li Lui at the Arizona Institute of Technology used pharmacogenomics, or the study of how genes interact with drugs, to solve the problem. The team searched for statistical associations between protein biomarkers, protein characteristics that indicate the therapy is working, as well as therapeutic agents and patient outcomes. A common pitfall of such models is over-fitting, finding too many biomarkers that are important statistically but not important biologically. The researchers corrected for over-fitting by adding more weight to certain biomarkers. In their model, they heavily prioritized clinical features and proteins that are highly conserved (Liu et al. 2017).
Why were highly conserved proteins important in the model? Highly conserved proteins were given more weight because they have important functions in the body. Conserved proteins by definition have few DNA mutations. Mutations tend to cause loss of function, and individuals who lose function of important genes are unable to survive. The death of those with harmful mutations contributes to a population with few mutations to important genes. In other words, those with functioning proteins have a survival advantage. Natural selection acted on unfit individuals to remove them and their harmful mutations from the population through natural selection. This is how evolution plays into this precision medicine model for AML.
The team also incorporated evolution into their model using Fitch’s Algorithm (Liu et al. 2017). Fitch’s Algorithm uses differences between different genomes to determine how different they are. The more distantly related two genomes are, the less likely the are to respond to the same AML treatment. Using the least differences principle stated above, the final weight attributed to each protein biomarker was the sum of the statistical weight and the evolutionary weight assigned by the researchers. Certain treatments were predicted to be successful in individuals with certain biomarkers.
To assess the power of their model, the team created other models and found that the high evolutionary weight from the combination of clinical and conserved sequences was a better model than either factor alone. The final model had an accuracy of 77.9%, which was the highest-performing among all the groups. They concluded that evolution can help define which markers are important in predicting the best treatment for individuals (Liu et al. 2017).
Questions
- Is there a better way to predict this?
- How are exactly are patients matched to a treatment?
- Which AML treatments are best for patients with particular biomarkers?
- What clinical studies need to be done next?
- How will the current political climate affect drug studies and efforts toward precision medicine put in motion by the previous administration?
- How can this method be incorporated efficiently into clinical practice?
- How will healthcare providers explain precision medicine to their patients?
Further Reading
From citizen science to Wikipedia, crowd-sourcing is not a new idea either. Crowdsourcing in science is thought of as a new form of citizen science. The term “citizen science’ was coined in 1995, but the first cases occurred in the 1800s (Droege 2007). The term was coined as recently as 2006 (Ellis 2014). The first documented case of crowdsourcing in science was in 2007 (Cook 2011), and since then it has become wildly popular in the world of science. To become one of many researchers, play a protein-folding videogame where players can model proteins used in human disease and contribute to scientific research of disease: https://fold.it/portal/info/about
Watch this funny video to learn about skill trees and balance updates in evolution: https://www.youtube.com/watch?v=m2FcWmo0sC0
Read more about crowdsourcing in science here: https://www.bostonglobe.com/ideas/2011/11/11/how-crowdsourcing-changing-science/dWL4DGWMq2YonHKC8uOXZN/story.html
This page provides details on the purpose and creation of DREAM Challenges. It lists several ongoing DREAM Challenges concerning medicine. Current challenges include analysis of multiple myeloma data, predicting transcription factor binding sites, and improving breast cancer detection using digital mammography. https://dreamchallenges.org/
Sage Bionetworks is a nonprofit that provides the platform and expertise behind the DREAM challenges. Its current projects include a comprehensive online cancer database, leading bioinformatics for teams of scientists who will improve personalized vaccines for cancer, and improving accuracy of digital mammography. https://sagebase.org/
The Acute Myeloid Outcome Prediction Challenge posting states the purpose of the challenge and lists objectives. The video on this page describes the creation of DREAM challenges, AML, the problem this challenge seeks to address, and information about analysis. https://www.synapse.org/#!Synapse:syn2455683/wiki/64007
References
- Cook, G. (2011). How crowdsourcing is changing science. The Boston Globe. Retrieved from https://www.bostonglobe.com/ideas/2011/11/11/how-crowdsourcing-changing-science/dWL4DGWMq2YonHKC8uOXZN/story.html
- Droege, S. (2007). Just because you paid them doesn’t mean their data are better. Citizen Science Toolkit Conference. Retrieved from https://www.birds.cornell.edu/citscitoolkit/conference/proceeding-pdfs/Droege%202007%20CS%20Conference.pdf
- Ellis, S. (2014). A History of Collaboration, a Future in Crowdsourcing: Positive Impacts of Cooperation on British Librarianship. Libri 2014, 64(1): 1−10. DOI: 10.1515/libri-2014-0001
- Kornblau, S., & Gustavo, S. (2014). DREAM 9 Challenge Launch. Retrieved from https://www.synapse.org/#!Synapse:syn2455683/wiki/64007
- Liu, L., Chang, Y., Yang, T., Noren, D.P., Long, B., Kornblau, S., Qutub., A., & Jieping, Y. (2017). Evolution-informed modeling improves outcome prediction for cancers. Evolutionary Applications, 10(1), 68-76. DOI: 10.1111/eva.12417
- National Institutes of Health. (2017). The Precision Medicine Initiative. U.S. National library of medicine. Genetics Home Reference. Retrieved from https://archive.is/GuBin
- National Institutes of Health. (2017). What is the difference between precision medicine and personalized medicine? U.S. National library of medicine. Genetics Home Reference. Retrieved from https://ghr.nlm.nih.gov/primer/precisionmedicine/precisionvspersonalized